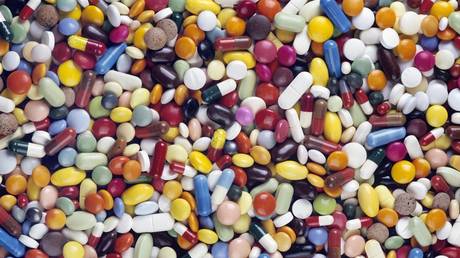
Scientists have used artificial intelligence to “predict” formulas for new designer drugs, with the stated goal of helping to improve their regulation. The AI generated formulas for nearly nine million potential new drugs.
Researchers with the University of British Columbia (UBC) used a deep neural net for the job, teaching it to make up chemical structures of potential new drugs. According to their study, released this week, the computer intelligence fared better at the task than the scientists had expected.
The research team used a database of known designer drugs – synthetic psychoactive substances – to train the AI on their structures. The market for designer drugs is ever-changing, since their manufacturers are constantly tweaking their formulas to circumvent restrictions and produce new “legal” substances, while cracking their structure takes months for law enforcement agencies, the researchers said.
“The vast majority of these designer drugs have never been tested in humans and are completely unregulated. They are a major public-health concern to emergency departments across the world,” one of the researchers, UBC medical student Dr. Michael Skinnider has said.
After its training, the AI was able to generate some 8.9 million potential designer drugs. Afterwards, researchers ran a data sheet of some 196 new drugs, which had emerged in real life after the model was trained, and found that more than 90% of these have been already predicted by the computer.
“The fact that we can predict what designer drugs are likely to emerge on the market before they actually appear is a bit like the 2002 sci-fi movie, Minority Report, where foreknowledge about criminal activities about to take place helped significantly reduce crime in a future world,” senior author Dr. David Wishart, a professor of computing science at the University of Alberta, has said.
Identifying completely unknown substances remains an issue for the AI, the research team has noted, but they hope it might potentially help with that task, since the computer was also able to predict which formulas of designer drugs were more likely to be created and hit the market. The model “ranked the correct chemical structure of an unidentified designer drug among the top 10 candidates 72 percent of the time,” while throwing in spectrometry analysis, which is an easily obtained measurement, bumped the accuracy to some 86%.
“It was shocking to us that the model performed this well, because elucidating entire chemical structures from just an accurate mass measurement is generally thought to be an unsolvable problem,” Skinnider stated.
Think your friends would be interested? Share this story!